ethical data-driven marketing in healthcare

in healthcare, the adoption of data-driven marketing technology looms as an evergreen discussion topic
The vast majority of these tools used by healthcare marketers today, many of which involve machine learning and artificial intelligence, are offered as part of broader marketing automation suites. For example, Send-Time Optimization (STO) tools are among the most widely adopted, which use machine-learning to allow marketers to send messages at a time that’s ideal for each subscriber. But STO is just the tip of the iceberg, as several not-so-startups are using a variety of machine learning approaches, notably natural language processing, to help marketers better connect with audiences. These applications power chatbots, analyze social media conversations, measure brand sentiment, streamline channel attribution, create specialized language and testing tools, and much, much more. Name a marketing channel or process, and there’s probably an AI-powered tool to help drive it.
And yet, as eager as many are to implement data-driven solutions, the application of these sophisticated tools is still in its infancy. As of April 2021, the adoption of these tools is still in its early stages — according to one large MarTech vendor, the adoption of AI tools in their marketing suite is ~5% of total users, and presumably even less in healthcare. But the ambition is clearly present, as evidenced by survey responders and the proliferation of firms aiming to develop AI and machine learning applications for any number of health system-related tasks. The burgeoning set of data-driven tools will be more contextual, rely on more complex and specific datasets, and will be centered around driving better care outcomes for patients and operational efficiencies for health systems. But as companies develop these models for use in driving patient insights and marketing communications, it’s important that these models and tools not fall prey to many of the pitfalls of models that have plagued earlier attempts at using machine learning to predict behavior in healthcare.
So how can health systems start thinking about ethical adoption and usage of data-driven marketing technologies? There are several considerations that health system executives and marketers should take into account as they make decisions about how to deploy machine learning and AI-enabled tools in their ecosystems.
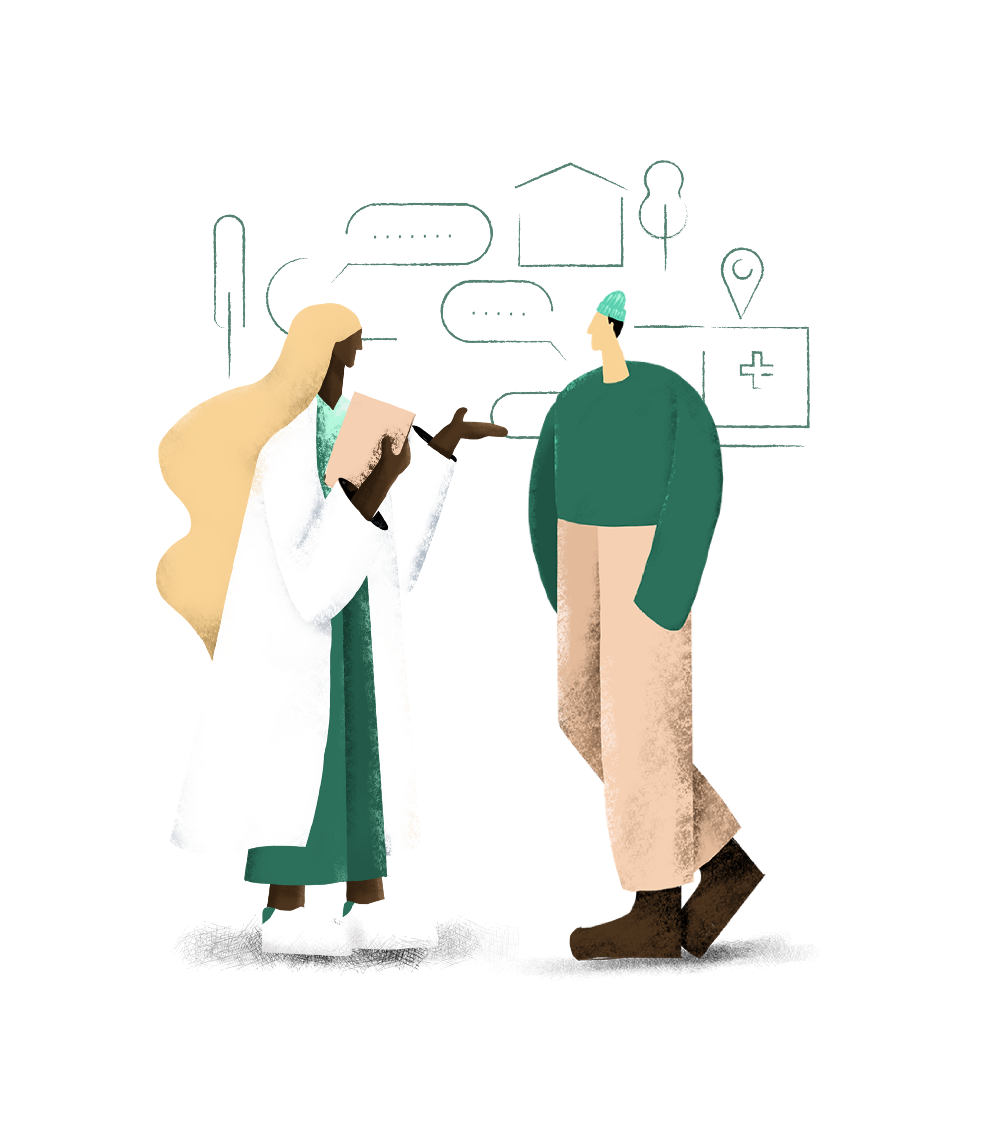
Artificial intelligence isn’t magic, however many companies in the market keep the specific decisions that are made at any given time hidden in a black box, and the data can be difficult to extract. To make intelligent decisions about models that are inherently complex and very technical, health system decision-makers should first ask critical questions when evaluating technology vendors. It may seem daunting, but it’s important that purchasers have a cursory understanding of what factors are most important and which factors are variable in machine learning tools, lest they risk any number of pitfalls, ranging from public relations crises to negative and discriminatory patient experiences.
To do this, decision-makers should engage individuals who can help them make intelligent evaluations of technology vendors and how their models are making decisions. This could come in the form of in-house data scientists, machine learning engineers, or third-party consultants, but as with any technology choice, health systems must perform their due diligence on all the tools they use on their patients, with marketing technology choices being no exception. In other words, health system buyers should apply the same due diligence to marketing automation tools as they do to complex medical devices — for marketing automation tools will arguably have more impact on patient outcomes (i.e., be a determining factor in whether or not the patient seeks care to begin with) than any individual physician or device.
The burden, however, isn’t entirely on health system decision-makers to decipher these complex solutions. Technology vendors should provide interpretable guides to explain how the tools that they build make decisions and how they proactively avoid or mitigate different kinds of bias, and then be accountable for the decisions made. There are several powerful incentives for technologists to do so: Not only will vendors who make it easier for lay audiences to understand the factors involved in decision making find their tools more widely adopted, these tools will then become better trained, and over time, better at making decisions. It’s a win-win.
In the next part of this series, we’ll dive into how Cured is working to make its data-driven solutions transparent. As technology providers, it’s incumbent on us to help our customers understand our technology, the algorithmic models that power it, and prescribe how best to use it. Even though several risks need to be thoughtfully evaluated, with the right tools and training, the next generation of data-driven solutions can create immense value for health systems and their patients.